

If len(A) = 0: return (IA,LOCB) if return_index else IA IA : #A 1D array, IA = True if and only if Return whether each row in A occurs as a row in B Here's a function based on libigl's igl::ismember_rows which closely mimics the behavior of Matlab's ismember(A,B,'rows'): def ismember_rows(A,B, return_index=False): Sb2 = np.expand_dims(bb,axis=1) + sb + np.expand_dims(bb,axis=1) Sa2 = sa2 + sa + np.expand_dims(ba,axis=1) Sa2 = np.expand_dims(ba,axis=1) + sa + np.expand_dims(ba,axis=1) Output unique numpy array with length m, storing either True or False, True for rows can be found in both A and B Input: m x n numpy array (A), and p x q array (B) The function first turns multiple columns of elements into a single column array, then numpy.in1d can be used This function is find which rows found in A can be also found in B, The function first turns multiple columns of elements into a single column array, then numpy.in1d can be used to find out the desire answer, please try the following code: import numpy as np Np.all(in1d_index(a2, b2) = ismember(a2, b2))Īs what unutbu said, the indices are returned in increasing order Return np.flatnonzero(np.in1d(b, a) & np.in1d(b, a)) So in1d_index is ~650x faster (for arrays of length in the low thousands), but again note the comparison is not exactly apples-to-apples since in1d_index returns the indices in increasing order, while ismember_rows returns the indices in the order rows of a show up in b. If the arrays are not contiguous, the data will be copied to a contiguous array, which will slow down the performance.ĭespite the caveats, one might choose to use in1d_index anyway for the sake of speed: def ismember_rows(a, b): asvoid works best on contiguous arrays.On float dtypes, since asvoid() = asvoid() returns asvoid will work for integer dtypes, but be careful if using asvoid.
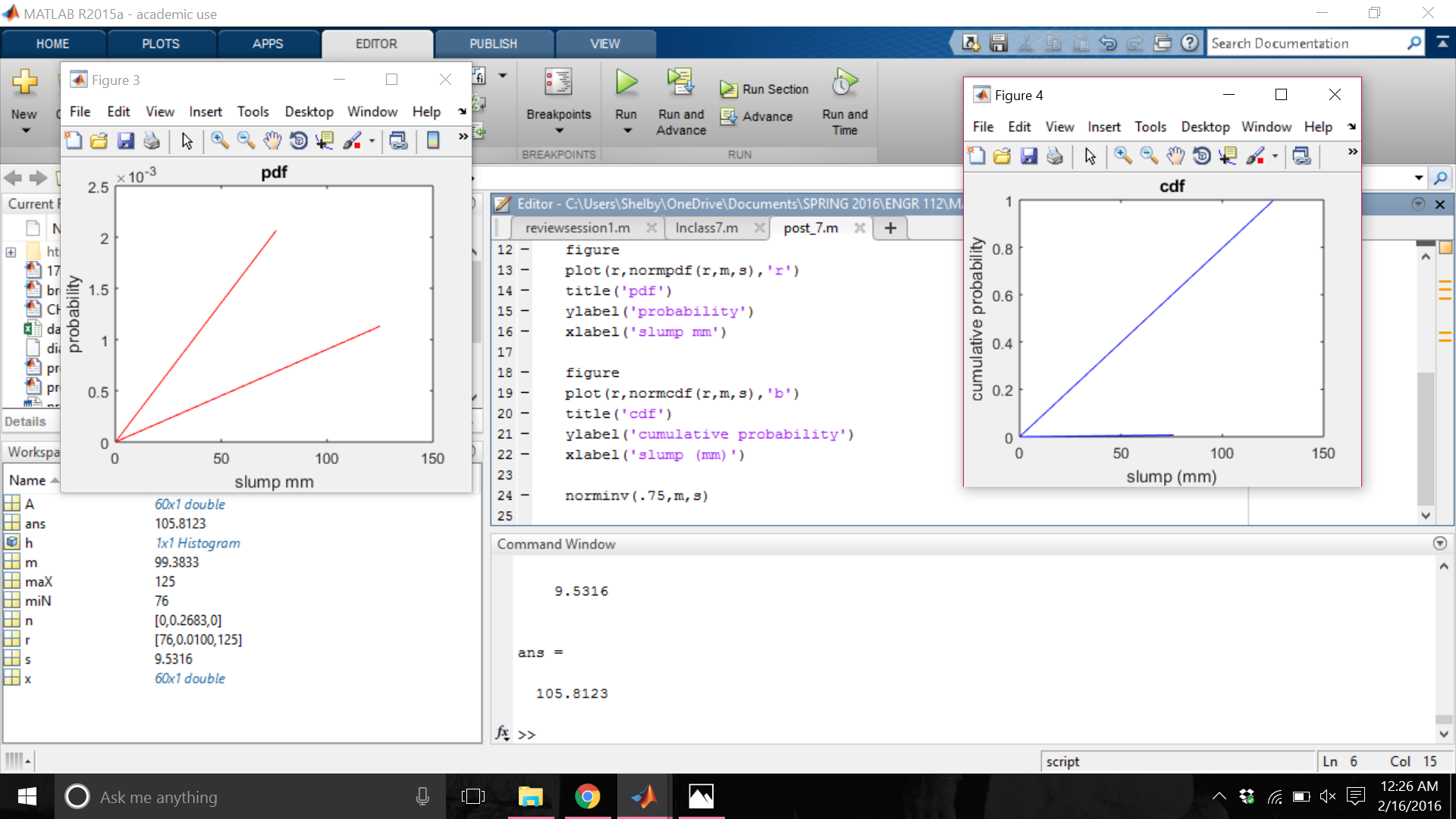
The indices are returned in increasing order.This would be equivalent to Matlab's, since Python uses 0-based indexing. Return np.where(np.in1d(voidb, voida))ī = np.array(,]) Return arr.view(np.dtype((np.void, * arr.shape))) Warning: When using asvoid for comparison, note that float zeros may compare UNEQUALLY This views the last axis of ND-arrays as bytes so you can perform comparisons on
